Submission declined on 23 April 2024 by Johannes Maximilian (talk). This submission is not adequately supported by reliable sources. Reliable sources are required so that information can be verified. If you need help with referencing, please see Referencing for beginners and Citing sources.
Where to get help
How to improve a draft
You can also browse Wikipedia:Featured articles and Wikipedia:Good articles to find examples of Wikipedia's best writing on topics similar to your proposed article. Improving your odds of a speedy review To improve your odds of a faster review, tag your draft with relevant WikiProject tags using the button below. This will let reviewers know a new draft has been submitted in their area of interest. For instance, if you wrote about a female astronomer, you would want to add the Biography, Astronomy, and Women scientists tags. Editor resources
| ![]() |
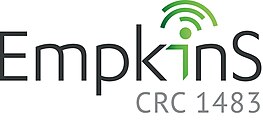
The Collaborative Research Centre (CRC) 1483 "EmpkinS" is located at the Friedrich-Alexander University Erlangen-Nuremberg and is funded by the German Research Foundation (DFG).[1][2] EmpkinS is an abbreviation for "Empatho-Kinaesthetic Sensor Technology – Sensor Techniques and Data Analysis Methods for Empatho-Kinaesthetic Modeling and Condition monitoring."
Speaker | Prof. Dr. -Ing. Martin Vossiek |
---|---|
Established | July 2021 |
Address | Carl-Thiersch-Straße 2b , 91052 Erlangen , Germany |
Website | https://www.empkins.de |
The focus of EmpkinS is capturing human motion parameters remotely in a minimally disturbing and non-invasive manner and with very high resolution. The physiological and behavioral states underlying the motion pattern are then reconstructed algorithmically from this data, using biomechanical, neuromotor, and psychomotor body function models. The sensors, body function models, and the inversion of mechanisms of action establish a link between the internal biomedical body layers and the outer biomedical technology layers. Research into this link is highly innovative and extraordinarily complex, and many of its facets have not been investigated so far.
To meet the numerous and diverse research challenges, the EmpkinS Collaborative Research Center is designed as an interdisciplinary research program. The coordinating university for the CRC is Friedrich-Alexander University Erlangen-Nuremberg. Scientists from the Hamburg University of Technology[3], the Technical University of Munich[4], and the Fraunhofer Institute for Integrated Circuits are also involved. The EmpkinS team consists of over 85 engineers, doctors, psychologists, and theologians from various fields.[5] The CRC 1483 is one of the most interdisciplinary Collaborative Research Centres in Germany.
Goal edit
EmpkinS aims to develop new digital diagnostic and therapeutic options in medicine and psychology, with the patient at the center. The future potential is based on the prospect of simultaneously recording multiple (dys)functions of the body using easily accessible and widely available sensors that are neither invasive nor burdensome. EmpkinS is working the expansion of currently available diagnostic medical technology, which is typically complex and costly, and primarily used as point-of-care technology in a doctor-centric approach, towards innovative, patient-centered, sensory, and diagnostic options.
Sub-projects edit
CRC EmpkinS consists of 19 subs-projects divided into 5 project areas.
Project Area A - Contactless sensors for recording motion parameters and viral functions edit
Project area A researches different wave and radio-based sensor technologies for the remote detection of motion parameters of the human body. The targeted new sensor concepts achieve a precision, measuring rate, dynamics and fine-grained resolution in all dimensions (6D pose, 3D body surface and 3D speed with the respective time course of all values) that are at least one order of magnitude better than the current state of the art are.
Coherently phase-sensitive, wave-based sensors such as radar, laser or coherent radio location systems are particularly suitable for measuring both macroscopic and microscopic movement processes remotely with maximum precision, as they are able to evaluate Doppler and micro-Doppler signal phases.
The EmpkinS sensor technologies are selected on the basis that as many different motion parameters as possible can be recorded with the highest possible quality. Since a single sensor cannot optimally record all conceivable parameters equally, complementary sensor technologies are being researched. They cover different types of detection areas (e.g. the entire body shell (sub project A01) or areas of interest such as the face, neck or arm, leg or chest area (A03, A04). Moreover different orders of magnitude of movement (e.g. movements of the limbs (A02) or microfasciculations (A05) e.g. on the face) and / or different areas of application (e.g. measuring vital parameters or the mobility of limbs) are recorded. Depending on the required input variables of the biomechanical neuro- and psychomotor models or depending on the diagnostic question, the different sensor technologies are used individually or in combination.
A01 Multimodal 3D Body Imaging Camera edit
Sub project A01 explores a novel, multimodal sensor concept for high-precision, non-contact detection of the envelope of the human body and the velocity vector of each point on this envelope. For this purpose, a microdoppler aperture synthesis radar is combined with an optical depth camera. The body envelope and its movements are detected with great precision and at a high measuring rate. With these properties, the system is a central basis for the research program of the CRC EmpkinS.
A02 Holographic 6D Wireless Locating and Motion Tracking edit
Sub project A02 is researching a radiolocation technique for high-precision tracking of human poses (3D position and 3D orientation of body parts). A novel, holographic extended Kalman filter makes it possible to evaluate the absolute signal phases of 61 GHz radio nodes, although the radio modules to be localized emit unsynchronized/inconsistent signals. This enables low-effort tracking with sub-millimetre measurement uncertainty and a measurement rate of up to 10 kHz, which opens up completely new possibilities for the detection of highly dynamic human movements.
A03 Highly Integrated Localizable Wireless EMG Transponder edit
In this sub project, localizable electromyography (EMG) radio transponders are to be designed and realized in order to be able to record surface EMG data synchronously with highly accurate radio localisation in real time for the first time. For this purpose, a 61-GHz transceiver in CMOS technology is being designed, which emits the phase-coherent signal required for the holographic radiolocation method and at the same time must be designed to be extremely energy-saving. In a further step, the transceiver is to be integrated into an EMG sensor platform, which is to be evaluated in test series on subjects, e.g. on the face or legs, to analyse facial expressions or gait.
A04 Microwave Interferometer for Cardiovascular and Respiratory Monitoring edit
The aim of this sub project is to research a microwave interferometric sensor that enables empatho-kinesthetic examinations based on the analysis of micro-movements of the body surface. Specifically, the temporal and morphological course of pulse waves, heart sounds and breathing of clothed persons is to be recorded in high resolution via relative distance measurements. The new circuit and algorithmic approaches will enable the recording of cardiovascular activity during simultaneous large-body movements and significantly improve the quality and thus the significance of the measured values.
A05 Electro-optic Microstructure- and Micromotion-Sensor edit
A novel approach for an electro-optical sensor is being researched that can image the smallest movements and changes (e.g. fasciculation or perspiration) of the skin surface without contact and with particularly high accuracy (< 100 nm) and frame rate (1 kHz) in an area of 1 cm^2 in real time. For this purpose, the state of the art is extended with simultaneous observation of 100 image points of a 2D image plane using digital signal processing and coherent lidar technology. The focus is on validating the sensor principle, first in the laboratory with phantoms, then in medical studies with D02–D05 to prove a diagnostic added value, e.g. to detect stress.
Project Area B - Sensor signal / data processing and transfer edit
Project Area B researches innovative methods and solutions for efficient sensor signal processing and transfer. All parts of the processing chain such as the sampling of the high-dimensional, continuous, physical signals, the efficient source coding of the scanned sensor values, the energy-saving mobile sensor data transfer and the visualization are addressed.
Specifically, the compressive sampling of 6D radar data is being researched in sub project B03 in order to shorten the recording time of empatho-kinaesthetic radar sensors. For this purpose, the common sparseness of the location and motion data is used and the radar test signals are optimized and adapted with regard to the compressive scanning. In B01 an efficient source coding method for multimodal body hull camera data is explored, which, similar to the mp3 format for audio data, enables efficient compressed transmission and storage of the human body hull data including it's motion. For this purpose, the spatial correlation of the depth information and the temporal coherence of the movement should be used in order to reduce the data volume by at least two orders of magnitude. Innovative protocols and algorithms for energy-efficient reference signal and electromyography (EMG) sensor data transmission are researched in B02. In order to meet the application-related, stringent requirements for energy efficiency, the joint design of reference and data signals as well as the integration of concepts for local energy generation are developed. Finally, B04 explores the visualization of motion sequences based on a biomechanical model. Derived states of the biomechanical model make the sensor signals easier to interpret for medical professionals, whereby visualizations should also be generated for patients that support communication. Important research aspects are the spatial registration and deformation of the multimodal sensor data and the biomechanical model as well as the integrated representation of these components.
B01 Coding of Multimodal Body Hull Data edit
The aim of the sub project is to develop a source coding method for multimodal body hull data that enables efficient transmission of the human body hull including its motion. The spatial correlation of the depth signals, the temporal coherence of the human motion and the inter-dependencies between microdoppler aperture synthesis radar data and depth signals are used for compression. Depending on the reconstruction accuracy of the biomechanical and psychomotor applications in the CRC, a reduction of the data volume by at least two orders of magnitude is expected.
B02 Protocols and Algorithms for Energy-efficient Reference Signal and EMG Sensor Data Transmission Integrating Local Energy Harvesting edit
Analytical and data-based models for the hardware components and signal processing blocks of the EmpkinS beacons and the base stations are developed to facilitate the design of resource allocation algorithms. Based on these models, optimization problems are formulated and their solutions form the basis for the derivation of resource allocation protocols and algorithms. The most promising resource allocation concepts are implemented, verified, and further refined in an experimental system.
B03 Compressive Sensing for Empatho-kinaesthetic Radar Sensors edit
Based on a synthetic data model as well as real measurement data provided by other subprojects, various methods for improving compressive sensing are investigated in order to reduce the measurement time of image-based radar technology in EmpkinS: This includes distributed compressive sensing, adaptive compressive sensing and radar test signals optimised for compressive sensing.
B04 Visualization of Motion Sequences Based in a Biomechanical Model edit
In sub project B04, innovative methods for the visualization of movement sequences that were recorded with various empatho-kinesthetic sensors and/or for which a biomechanical simulation was carried out are being researched. The focus is on visualizations and the extraction of features that support medical interpretation and diagnosis.
Project Area C - Biomechanical modeling and conditioning edit
Project area C explores approaches to process, model and interpret biomechanical data. This is an integral part of EmpkinS in order to obtain meaningful movement information on the basis of the large amount of available measurement data, which originate from innovative measurement principles and therefore entail completely new requirements, and thus to be able to carry out further analysis.
In detail, musculoskeletal human models are improved in C01 by personalization using machine learning approaches. This will help to distinguish individual differences in motion characteristics from those that are caused, for example, by neurological or psychological conditions in general. In C02, musculoskeletal human models are used to filter and analyse measurement data by relating the measurement data to the kinematic / dynamic motion capabilities of the human musculoskeletal system. The aim of C03 is then to use the (improved) models and (adjusted) measurement data to investigate a new model of postural control of standing and walking. This model uses measurements of the empatho-kinaesthetic sensory system and a sensorimotor-enhanced musculoskeletal human model to characterize the components of dynamic balance control and to advance research into balance regulation. Finally, in C04, the analysis and prediction of biomechanical simulation models are improved by integrating empatho-kinaesthetic sensor data. This is achieved by integrating measurement data (position, orientation, speed, acceleration, force, muscle activity) into the mathematical formulation of a motion process as an optimal control problem. The selection of the model approaches is based on the requirement to research and provide the highest possible quality analysis options for the consideration of EmpkinS.
C01 Machine Learning for Personalization of Musculoskeletal Models, Movement Analysis and Movement Predictions edit
The extent to which machine learning can be used to effectively personalize gait simulations using motion data is explored. We first investigate the influence of body parameters on gait simulations. An initial version of the personalization is trained with simulated motion data, since ground truth data is known for this purpose. We then explore gradient-free methods to fit the network for experimental motion data. The resulting network is validated with magnetic resonance imaging, electromyography and intra-body variables.
C02 Filtering of Multimodal Motion Capture Data using Individualized Musculoskeletal Human Models edit
Novel methods for analyzing and filtering multimodal motion measurement data in conjunction with individualized musculoskeletal full-body models in multiple domains are being researched. Based on the individualized muscoskeletal models, kinematic and dynamic tracking algorithms are investigated that can be applied to different combinations of multimodal measurement data (e.g. position, orientation and surface data). Furthermore, the newly developed methods will be tested and evaluated, and benchmarked against conventional methods. Novel EmpkinS measurement technology will be transferred to the developed methods as soon as they are available.
C03 Investigation of Postural Control using Sensomotor Enhanced Musculoskeletal Human Models edit
A novel postural control model of standing and walking is explored to characterize the components of dynamic balance control. For this purpose, clinically annotated motion data is used as input and muscle actuated multi-body models are enhanced by a sensorimotor level. Neuromotor and control model parameters of (patho-)physiological movement are identified via machine learning methods. Technical and clinical validation of the models will be performed. New EmpkinS measurement techniques are to be transferred to the developed models.
C04 Analysis of Degenerative Motion Impairments through Integration of Empatho-kinaesthetic Sensor Data in Biomechanical Human Models edit
The focus of this project is on the best possible integration of empatho-kinesthetic sensor data into biomechanical models. Specifically, degenerative movement restrictions of the hand are recorded by EmpkinS and reference sensor technology and the data are optimally integrated into the mathematical formulation of the optimal control problem depending on data type, measurement frequency, data accuracy, etc. The aim of the project is to develop a model of healthy and of degenerative hand movement. Objective biomarkers of healthy or impaired movement function are identified through movement tracking and prediction.
Project Area D - Physiological and behavioral modeling and condition monitoring edit
In project area D, sensor technologies and empatho-kinaesthetic procedures are researched on various diseases and internal states. Important aspects in this project area are the research of medical and psychological body function models for the transformation of the sensor-based EmpkinS measured variables into clinically relevant parameters and the research into new forms of diagnosis and therapy that are based on these parameters and the EmpkinS body function models. The overriding goal is to assign a medical or psychological relevance to the sensory parameters. In order to achieve this, the sub projects focus on different (patho-) physiological states. These states are characteristic of the disease models examined and include prototypically disturbed body functions, whereby the applicability of the different methods is to be demonstrated.
The clinical aspects considered in project D area are hand function in rheumatic diseases (D01), facial expressions, posture and movement in depressed patients (D02) and, stress-associated, pathophysiological changes in the skin, cardiopulmonary function, facial expressions, general body movement and the detection of microfasciculation (D03). Furthermore, gross motor skills and cardiovascular changes are addressed in D04 as a measure of sleep and movement disorders in Parkinson’s disease, while D05 is devoted to the investigation of body movements and cardiopulmonary function for the assessment of palliative patients in regard to their wellbeing.
In this way, the perspective transferability of the EmpkinS methodology is evaluated using a broad spectrum of body functions selected as an example: from fine motor skills to gross motor skills and cardiovascular function to the evaluation of psychological functions such as depression and stress reactions. At the same time, different areas of application of medicine are being researched, from diagnostics (D01, D03–D05) to intervention (D02, D04) to prognosis, care and supply support (D04, D05). In the sub projects it is demonstrated that the empatho-kinaesthetic parameters can depict functional disorders and are therefore a measure of (patho-) physiological processes.
D01 Hand Motion Patterns derived from Empatho-kinaesthetic Sensor Data as a Diagnostic Parameter for Disease Activity in Patients with Rheumatic Diseases edit
The aim of D01 ist to characterize hand motion in patients with inflammatory arthritis and to investigate the relation of hand motion with disease activity. A comprehensive data set comprising disease status of patients (N = 150) with rheumatoid arthritis (RA) and psoriatic arthritis (PsA) clinical hand function assessments and state-of-the-art motion capture analysis of hand motion provides a critical basis for the experimental evaluation and integrated data interpretation of EmpkinS data (together with sub projects A01, A02 and A03). Patient data will be compared with a healthy cohort (N = 75).
D02 Empatho-kinaesthetic Sensor Technology for Biofeedback in Depressed Patients edit
The aim of the D02 project is the establishment of empatho-kinesthetic sensor technology and methods of machine learning as a means for the automatic detection and modification of depression-associated facial expressions, posture, and movement. The aim is to clarify to what extent, with the help of kinaesthetic-related modifications influence depressogenic information processing and/or depressive symptoms. First, we will record facial expressions, body posture, and movement relevant to depression with the help of currently available technologies (e.g., RGB and depth cameras, wired EMG, established emotion recognition software) and use them as input parameters for new machine learning models to automatically detect depression. Secondly, we will develop and validate an intervention in which facial expressions are used to validate antidepressive self-statements and invalidate depressogenic self-statements. Findings from this trial will help clarify whether the efficacy of cognitive interventions against depression can be further enhanced by systematically targeting kinesthetic information processing. Finally, we will explore possibilities of integrating this new intervention in mobile-based treatments for depression.
D03 Contact-free Measurements of Stress, its Determinants and Consequences edit
The aim of this sub project is to make stress measurable in a contact-free way using empatho-kinesthetic sensor technology. Due to its effects on human health and performance, research into stress has a high priority, but is hampered by costly and mostly invasive traditional recording methods. In D03, empatho-kinesthetic sensory modalities are being researched in laboratory experimentally induced, acute stress situations, which make it possible to make stress measurable through contactless recording of macro- and micro-movements in collaboration with A02, A04 and A05.
D04 Sensor-based Movement and Sleep Analysis in Parkinson's Disease edit
D04 evaluates innovative, non-contact EmpkinS sensor technology using machine learning algorithms and multimodal reference diagnostics and is aplying it to Parkinson’s-associated sleep disorder and its specific patterns. For this purpose, physiological parameters of sleep are validated technically with wearable sensor technology and non-contact EmpkinS sensor technology in comparison to classical poly-somnography and psychometric clinical scales. In an algorithmic approach, multiparametric sleep parameters and sleep patterns are then evaluated in correlation to movement, cardiovascular and sleep disorders.
D05 Empatho-kinaesthetic Assessment and Pattern Recognition of Movement as Biomarkers for Health Status, Well being and Prognosis of Palliative Patients edit
For the first time, D05 is investigating movement as a biomarker, i.e. objectifiable, measurable and quantifiable parameters that have diagnostic and predictive significance for the state of health, well-being and prognosis of palliative patients. These biomarkers are examined with patients in the laboratory and living lab of the palliative care unit. Contactless sensor technology allows scientific access to the last phase of life for the first time. Similarly, D05 is researching the sociological challenges of medical technology innovations in palliative care as a prototype for all health care sectors.
Project Area E - Ethics in and by Design edit
Project area E researches the ethical, legal and social issues in the context of EmpkinS and develops orientation marks for its future societal design. In the sense of an “ethics in and by design” approach, the aim is not only to critically accompany technological development, but also to sound out and implement specific design and decision paths. When researching the normative core concepts, the focus is on the concepts of human dignity, informational self-determination, justice and solidarity in times of big data and research and applications driven by algorithms. Furthermore, in this field persistent social attitudes, values and their impact on emerging technologies e. g. new AI-driven sensor technologies are researched. Not least are E is addressing this issue in constant dialogue with different stakeholders from society and put much emphasis on also engaging the broader public in the research.
Weblinks edit
References edit
- ^ "EmpkinS - ein neuer Sonderforschungsbereich für die FAU und das Department EEI". Department Elektrotechnik-Elektronik-Informationstechnik (in German). 2021-05-25. Retrieved 2024-04-23.
- ^ "DFG - GEPRIS - SFB 1483: Empathokinästhetische Sensorik – Sensortechniken und Datenanalyseverfahren zur empathokinästhetischen Modellbildung und Zustandsbestimmung". gepris.dfg.de. Retrieved 2024-04-23.
- ^ "ET3: EmpkinS". www.tuhh.de. Retrieved 2024-04-23.
- ^ "EmpkinS". www.ee.cit.tum.de (in German). Retrieved 2024-04-23.
- ^ "People". EmpkinS. Retrieved 2024-04-23.